Yes to AI, but basics first
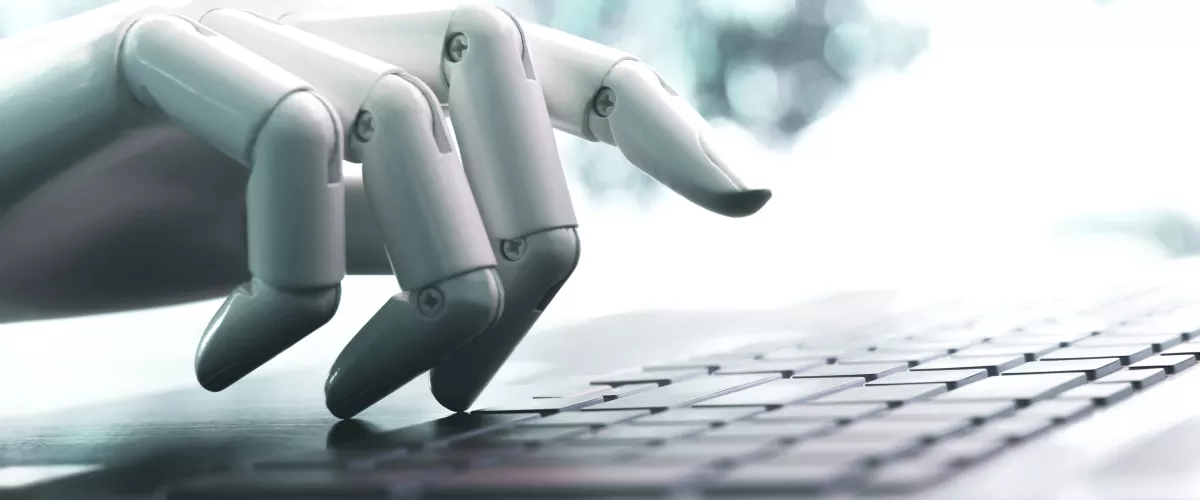
by Nephy PEREZ, Senior Vice President, Innovation and Supply Chain Solutions, Toll Group
When it comes to generative AI, are we sprinting before we can walk?
Over the first half of 2023, another epidemic has taken the world by storm, one the Wall Street Journal terms the “ChatGPT fever.” But a recent Salesforce study has found that while three in five people already use generative AI at work, a larger percentage of leaders don’t think their organisations are ready to use it or know how to get the most value out of it.
That is not to say we should stop exploring the incredible opportunities generative AI offers. Rather, it’s a call for a more systematic approach. Before we can tap into the full potential of large language models and machine learning, we need to first invest in a strong foundation for our people, processes, and technology.
And the urgency is real – properly utilized, generative AI can increase productivity by 40-60% according to most conservative estimates. What if your teams could increase their speed and quality by 40-60%? What if your competitors could?
While generative AI can write essays, generate code, and even compose music, it can't prepare our businesses to use it. That's a lesson we must learn ourselves.
A potential game-changer for supply chains
While AI is a very broad term, excitement has exploded over a specific facet: generative AI. This refers to artificial intelligence models that can generate high-quality outputs, such as text, images, and even simulations, based on existing data. Recent advancements in neural network algorithms, computational power, and availability of large datasets have made generative AI more accessible, versatile, and effective than ever before.
This means generative AI models can operate across the spectrum of being descriptive, predictive, and prescriptive. Not only can systems describe what’s happening now, they can also predict future outcomes and provide recommendations on how to achieve desired results. This empowers businesses to make more informed and strategic decisions, especially in their logistics operations and supply chains.
For one, generative AI can dramatically improve demand forecasting and inventory optimisation. Retail companies can simulate inventory strategies based on past sales data, social media trends, and even the weather to reduce stockouts and inventory costs.
Generative AI can also design better supply chains. Whether it’s how warehouse workers should move between racks or how goods should be shipped from warehouse to customer, generative AI can optimize routes based on locations, tariffs, fuel costs, traffic, and even natural disasters. With a full view of shipments and inventory levels, systems can predict that cargo arrival might be later than expected and recommend expedited deliveries from another location to avoid stockouts during sale season.
It also poses a time-saving alternative for the laborious part of any supply chain management: documentation. Generative AI-enabled tools can drastically reduce time spent on administrative tasks, such as creating commercial invoices, reviewing customs documents, or comparing contract terms across multiple suppliers for negotiation.
But AI isn't a miracle worker that you can just plug into a system and expect radical results.
Before integrating generative AI, businesses must make sure they have the basics in place to leverage its full potential. These are some of the key lessons we’ve learnt while applying AI for supply chains at Toll.
Define the business objective and required data
First, it is essential to define a clear objective — what goal is the company hoping to achieve through AI? Without one, even the most advanced generative AI system will become a dusty tool in the shed: underutilized and undervalued.
As we deploy applications at Toll using our in-house integrated AI platform, we focus on identifying “golden” use cases across the whole supply chain – those that can create the largest impact relative to existing, best-in-class solutions. Whether the goal is to optimize supply chain efficiency or personalize customer experience, success must be clearly defined.
Instead of rushing to an AI-enabled solution, we first ask three questions: What is the cost of not solving this problem today? Is it a problem that machine learning models can help to solve? What data is required to train these models effectively?
The third question assesses another critical factor for generative AI: data. More specifically, accurate, accessible, complete, and timely data. Since AI thrives on data, we’ve consistently seen that the most sophisticated models are only as good as the data they’re trained on.
Start by doing a stock take of your company’s data infrastructure. Is there a governance framework to ensure the data required for this business problem is reliable, up-to-date, and comprehensive? Are there data silos that hinder accessibility and completeness? Simply by helping customers centralize inventory and transport data, we’ve identified opportunities for operational performance and sustainability. Additionally, governance measures should prevent sensitive data leaks and safeguard privacy, aided by our use of in-house AI models.
Timeliness and accuracy can often be challenges in logistics, especially with manual data collection and input. This highlights the imperative for a connected supply chain, one that is integrated from truck to ship to storage. Connected platforms can provide more visibility through less touchpoints. Internet of Things (IoT) sensors can provide real-time monitoring, ensuring current and accurate data for AI models.
Equip people with more than tools
Beyond technology and data, people remain the key to success for generative AI to succeed. Successfully implementing AI-driven tools hinges on having a workforce that values AI’s potential in augmenting rather than replacing capabilities. Communication, training, and support mechanisms are vital to ensure that employees are equipped not just with what to use, but also when to use it.
At Toll, we’ve established multidisciplinary teams of data scientists, AI engineers, and business leaders to marry what’s needed with what’s possible. And in the face of an ever-evolving AI landscape, we consistently find ourselves needing to learn and upskill in data analytics and machine learning. Emerging technologies mean that what works today may not be sufficient tomorrow.
Above all, a willingness to experiment, fail, learn, and try again is pivotal. Using AI effectively is a continuous cycle of adapting and evolving, one that favours starting small and growing over time rather than immediately expecting all the right answers. Just as Google wasn’t the first search engine, the best use of generative AI might have yet to arrive.
Generative AI holds immense promise for transforming businesses, logistics, and supply chains, but it’s not a miracle worker. Like any tool, its effectiveness depends on the skill and preparedness of those who wield it. Building a foundation of clear objectives, data quality, and an equipped workforce helps us walk so that eventually, we can sprint.
---
This article was published in The Business Times on 11 October 2023: https://www.businesstimes.com.sg/opinion-features/yes-ai-basics-first